Pandas Groupby: Summarising, Aggregating, and Grouping data in Python
Last Updated :
29 Aug, 2022
GroupBy is a pretty simple concept. We can create a grouping of categories and apply a function to the categories. It’s a simple concept, but it’s an extremely valuable technique that’s widely used in data science. In real data science projects, you’ll be dealing with large amounts of data and trying things over and over, so for efficiency, we use Groupby concept. Groupby concept is really important because of its ability to summarize, aggregate, and group data efficiently.
Summarize
Summarization includes counting, describing all the data present in data frame. We can summarize the data present in the data frame using describe() method. This method is used to get min, max, sum, count values from the data frame along with data types of that particular column.
- describe(): This method elaborates the type of data and its attributes.
Syntax:
dataframe_name.describe()
- unique(): This method is used to get all unique values from the given column.
Syntax:
dataframe['column_name].unique()
- nunique(): This method is similar to unique but it will return the count the unique values.
Syntax:
dataframe_name['column_name].nunique()
- info(): This command is used to get the data types and columns information
Syntax:
dataframe.info()
- columns: This command is used to display all the column names present in data frame
Syntax:
dataframe.columns
Example:
We are going to analyze the student marks data in this example.
Python3
# importing pandas as pd for using data frame
import pandas as pd
# creating dataframe with student details
dataframe = pd.DataFrame({'id': [7058, 4511, 7014, 7033],
'name': ['sravan', 'manoj', 'aditya', 'bhanu'],
'Maths_marks': [99, 97, 88, 90],
'Chemistry_marks': [89, 99, 99, 90],
'telugu_marks': [99, 97, 88, 80],
'hindi_marks': [99, 97, 56, 67],
'social_marks': [79, 97, 78, 90], })
# display dataframe
dataframe
Output:

Python3
# describing the data frame
print(dataframe.describe())
print("-----------------------------")
# finding unique values
print(dataframe['Maths_marks'].unique())
print("-----------------------------")
# counting unique values
print(dataframe['Maths_marks'].nunique())
print("-----------------------------")
# display the columns in the data frame
print(dataframe.columns)
print("-----------------------------")
# information about dataframe
print(dataframe.info())
Output:
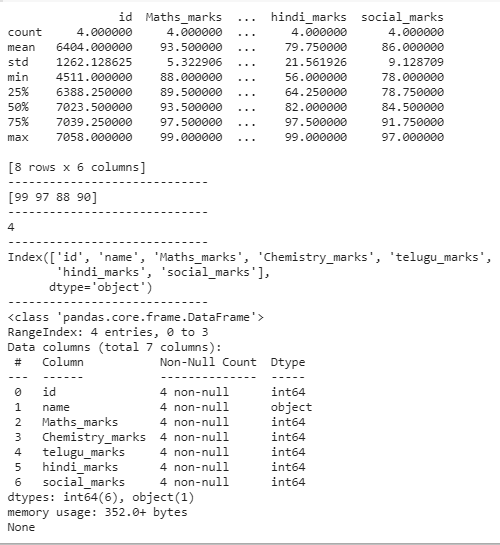
Aggregation
Aggregation is used to get the mean, average, variance and standard deviation of all column in a dataframe or particular column in a data frame.
- sum(): It returns the sum of the data frame
Syntax:
dataframe['column].sum()
- mean(): It returns the mean of the particular column in a data frame
Syntax:
dataframe['column].mean()
- std(): It returns the standard deviation of that column.
Syntax:
dataframe['column].std()
- var(): It returns the variance of that column
dataframe['column'].var()
- min(): It returns the minimum value in column
Syntax:
dataframe['column'].min()
- max(): It returns maximum value in column
Syntax:
dataframe['column'].max()
Example:
In the below program we will aggregate data.
Python3
# importing pandas as pd for using data frame
import pandas as pd
# creating dataframe with student details
dataframe = pd.DataFrame({'id': [7058, 4511, 7014, 7033],
'name': ['sravan', 'manoj', 'aditya', 'bhanu'],
'Maths_marks': [99, 97, 88, 90],
'Chemistry_marks': [89, 99, 99, 90],
'telugu_marks': [99, 97, 88, 80],
'hindi_marks': [99, 97, 56, 67],
'social_marks': [79, 97, 78, 90], })
# display dataframe
dataframe
Output:

Python3
# getting all minimum values from
# all columns in a dataframe
print(dataframe.min())
print("-----------------------------------------")
# minimum value from a particular
# column in a data frame
print(dataframe['Maths_marks'].min())
print("-----------------------------------------")
# computing maximum values
print(dataframe.max())
print("-----------------------------------------")
# computing sum
print(dataframe.sum())
print("-----------------------------------------")
# finding count
print(dataframe.count())
print("-----------------------------------------")
# computing standard deviation
print(dataframe.std())
print("-----------------------------------------")
# computing variance
print(dataframe.var())
Output:

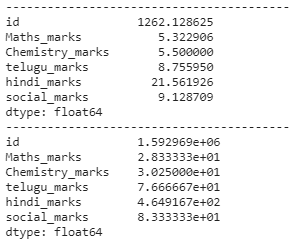
Grouping
It is used to group one or more columns in a dataframe by using the groupby() method. Groupby mainly refers to a process involving one or more of the following steps they are:
- Splitting: It is a process in which we split data into group by applying some conditions on datasets.
- Applying: It is a process in which we apply a function to each group independently
- Combining: It is a process in which we combine different datasets after applying groupby and results in a data structure
Example 1:
Python3
# importing pandas as pd for using data frame
import pandas as pd
# creating dataframe with student details
dataframe = pd.DataFrame({'id': [7058, 4511, 7014, 7033],
'name': ['sravan', 'manoj', 'aditya', 'bhanu'],
'Maths_marks': [99, 97, 88, 90],
'Chemistry_marks': [89, 99, 99, 90],
'telugu_marks': [99, 97, 88, 80],
'hindi_marks': [99, 97, 56, 67],
'social_marks': [79, 97, 78, 90], })
# group by name
print(dataframe.groupby('name').first())
print("---------------------------------")
# group by name with social_marks sum
print(dataframe.groupby('name')['social_marks'].sum())
print("---------------------------------")
# group by name with maths_marks count
print(dataframe.groupby('name')['Maths_marks'].count())
print("---------------------------------")
# group by name with maths_marks
print(dataframe.groupby('name')['Maths_marks'])
Output:

Example 2:
Python3
# importing pandas as pd for using data frame
import pandas as pd
# creating dataframe with student details
dataframe = pd.DataFrame({'id': [7058, 4511, 7014, 7033],
'name': ['sravan', 'manoj', 'aditya', 'bhanu'],
'Maths_marks': [99, 97, 88, 90],
'Chemistry_marks': [89, 99, 99, 90],
'telugu_marks': [99, 97, 88, 80],
'hindi_marks': [99, 97, 56, 67],
'social_marks': [79, 97, 78, 90], })
# group by name
print(dataframe.groupby('name').first())
print("------------------------")
# group by name with social_marks sum
print(dataframe.groupby('name')['social_marks'].sum())
print("------------------------")
# group by name with maths_marks count
print(dataframe.groupby('name')['Maths_marks'].count())
Output:

Similar Reads
Grouping and Aggregating with Pandas
When working with large datasets it's used to group and summarize the data to make analysis easier. Pandas a popular Python library provides powerful tools for this. In this article you'll learn how to use Pandas' groupby() and aggregation functions step by step with clear explanations and practical
3 min read
Groupby without aggregation in Pandas
Pandas is a great python package for manipulating data and some of the tools which we learn as a beginner are an aggregation and group by functions of pandas. Groupby() is a function used to split the data in dataframe into groups based on a given condition. Aggregation on other hand operates on se
4 min read
Grouping Categorical Variables in Pandas Dataframe
Firstly, we have to understand what are Categorical variables in pandas. Categorical are the datatype available in pandas library of python. A categorical variable takes only a fixed category (usually fixed number) of values. Some examples of Categorical variables are gender, blood group, language e
2 min read
Aggregation in MongoDB using Python
MongoDB is free, open-source,cross-platform and document-oriented database management system(dbms). It is a NoSQL type of database. It store the data in BSON format on hard disk. BSON is binary form for representing simple data structure, associative array and various data types in MongoDB. NoSQL is
2 min read
How to combine Groupby and Multiple Aggregate Functions in Pandas?
Pandas is an open-source Python library built on top of NumPy. It allows data structures and functions to manipulate and analyze numerical data and time series efficiently. It is widely used in data analysis for tasks like data manipulation, cleaning and exploration. One of its key feature is to gro
3 min read
Pyspark GroupBy DataFrame with Aggregation or Count
Pyspark is a powerful tool for working with large datasets in a distributed environment using Python. One of the most common tasks in data manipulation is grouping data by one or more columns. This can be accomplished using the groupBy() function in Pyspark, which allows you to group a DataFrame bas
3 min read
Pandas Cheat Sheet for Data Science in Python
Pandas is a powerful and versatile library that allows you to work with data in Python. It offers a range of features and functions that make data analysis fast, easy, and efficient. Whether you are a data scientist, analyst, or engineer, Pandas can help you handle large datasets, perform complex op
15+ min read
Python | Pandas dataframe.aggregate()
Dataframe.aggregate() function is used to apply some aggregation across one or more columns. Aggregate using callable, string, dict or list of string/callables. The most frequently used aggregations are: sum: Return the sum of the values for the requested axismin: Return the minimum of the values fo
2 min read
Append list of dictionary and series to a existing Pandas DataFrame in Python
In this article, we will discuss how values from a list of dictionaries or Pandas Series can be appended to an already existing pandas dataframe. For this purpose append() function of pandas, the module is sufficient. Syntax: DataFrame.append(other, ignore_index=False, verify_integrity=False, sort=N
2 min read
Create Grouped Bar Chart using Altair in Python
Grouped bar charts are a handy tool to represent our data when we want to compare multiple sets of data items one against another. To make a grouped bar chart, we require at least three rows of three columns of data in our dataset. The three columns can be used as- one for values, one for series, an
3 min read